Agriculture is an activity that is constantly evolving. Improvements, technologies, and farmer training show us that agriculture has always been a field of evolution. Contrary to what many people imagine, farmers are active researchers because they are the ones who experience the entire production process. In this way, they are able to perceive the efficiency of different management methods because they are always working with experiments, testing new seeds, fertilizers, pesticides, different types of management, and the use of technologies from the so-called agriculture 4.0, better known as digital agriculture.
To produce food sustainably and meet the growing demands of the population, which is expected to grow by more than 2 billion by 2050, the agriculture of the future needs to increase productivity, extracting maximum value from each stage of the production cycle. The production process must integrate agronomic knowledge, large agricultural databases (Big Data), innovative sensor technologies, satellites, unmanned aerial vehicles, autonomous machines and robots, software and cloud platforms (in general, the availability of computational resources, especially storage and processing, which dispense with the direct active management of the end user) (BASSOI et al., 2019).
Thanks to the use of available technologies, it is possible to increase production per area without increasing the planted area, avoiding the opening of areas intended for environmental preservation, using agricultural inputs in correct and efficient quantities for production, avoiding losses that could cause damage to the environment and thus, increasingly opening the vision that sustainable agriculture is an ally of agriculture 4.0.
For Bassoi et al. (2019), automation in rural areas is a reality. It occurs in all stages of production systems (preparation and planting, harvesting, crop management, processing, etc.) with the aim of increasing productivity; optimizing the use of time, inputs, and capital; reducing production losses; increasing product quality; and improving the quality of life of rural workers. However, the introduction of new concepts of precision agriculture and digital age tools, with great connectivity and integration of sensors and data, has generated new demands both in terms of new devices and systems integration, new methods, and protocols. Due to the complexity of agricultural production processes, which include biological, environmental, and socioeconomic aspects, automation constitutes a frontier with enormous opportunity for advancement.
In order to expand knowledge, in this text we will address some topics that are related to the advancement of agriculture, such as: precision agriculture, the use of drones and digital agriculture.
Precision agriculture
Precision agriculture is a management technique that considers spatial variability and allows the site-specific application of inputs, such as fertilizers, correctives, pesticides, seeds, water, and others. Also considering temporal variability, precision agriculture allows a more rational use of inputs, at the correct time, place, and dose, with potential economic and environmental benefits (EZENNE et al. 2019). Since precision agriculture is a production management and management system highly dependent on field data and information (plants, animals, soil, climate, machines, etc.), georeferenced, digitized, and with high flow, it provides a structural and conceptual basis for connecting agricultural production systems to the digital world, opening a channel for collecting and sharing data from the field (BASSOI et al., 2019).
Precision agriculture can be understood as a cycle that begins with data collection, analysis and interpretation of this information, generation of recommendations, application in the field and evaluation of results (GEEBERS; ADAMCHUK, 2010). Thus, precision agriculture is a tool that assists producers in making managerial decisions in crop management, taking into account the spatial and temporal variability of the crop to obtain maximum economic return and reduce environmental impact (INAMASUET al., 2011). It is, therefore, a chain of knowledge, in which machines, devices, equipment and software are tools for data collection, which must be organized and interpreted, generating information to support management (INAMASU; BERNARDI, 2014).
The contribution of precision agriculture tools to the sustainability of agricultural systems goes beyond mapping input needs, production and nutrient extraction. They can also contribute to conservation planning, as shown by Berry et al. (2003; 2005). The authors demonstrated soil and water conservation through the use of integrated spatial technologies (GPS, remote sensing and Geographic Information System – GIS), which assist in the analysis of spatial and temporal relationships for a better understanding of how these systems work. In this way, it would be possible to select management practices that maximize crop yields and quality, while simultaneously optimizing the use of inputs and reducing soil and water losses in agricultural areas (DELGADO; BERRY, 2008). And this can be achieved by determining altimetry, establishing water flows and areas at risk of erosion.
The application of inputs at variable rates has been the main form of use of precision agriculture tools in crop management (INAMASU; BERNARDI, 2014). Figure 1 shows a map of phosphate fertilization recommendations established from georeferenced soil samples. It is observed that the application of fertilizers without considering spatial variability can lead to negative environmental and economic impacts and, thus, compromise the sustainability of the agricultural production system (SANTOS et al., 2017; BERNARDI et al., 2016).
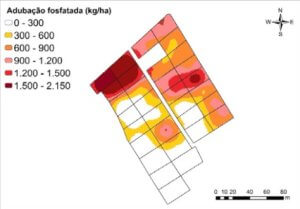
Automation is a system in which operational processes in agricultural, livestock and/or forestry production are monitored, controlled and executed by means of machines and/or mechanical, electronic or computational devices to increase human work capacity (INAMASUET al., 2016). Precision agriculture can also be cited as a successful case of automation. Many devices used in precision agriculture are agricultural equipment and machines with extensive use of electronics and digital systems (BASSOI et al., 2019)..
Digital agriculture/ smart agriculture/ smart farm
The literature on smart agriculture (ai) or smart farm (sf) is recent. Rapid developments in the internet of things (IoT) (VERDUM et al., 2016) and cloud computing are driving smart agriculture (SUND-MAEKERET et al., 2016) or digital agriculture (BASSOI et al., 2019). The basis for advancement in this sector involves a combination of internet technologies and technologies oriented towards the use of smart objects (BRETTELET al., 2014; LIAO et al., 2017).
However, if precision agriculture considers variability in the field, smart agriculture goes further, as it establishes management actions not only based on the location of the field, but also on stored data, reinforced by the context and situation of the crop, and also fed by data collected in real time (WOLFERT; SØRENSEN; GOENSE, 2014). Thus, there are interfaces and technologies that overlap and encompass ideas such as precision agriculture and management information systems in agriculture, which were derived from the idea of the Farm Management Integrated System (FMIS). The FMIS was defined by Sørensen et al. (2010) as a system designed to collect, process, store and disseminate data in a standardized format to perform operations and functions on rural properties. Smart agriculture incorporates information and communication technologies into machines, equipment and sensors in agricultural production systems, and allows the generation of a large volume of data and information with progressive insertion of automation in the process (PIVOTO et al., 2017).
The use of smart agriculture tools is possible due to sensors developed for use in agriculture. According to Lehmann et al. (2012), a sensor is an electrotechnical device that responds to a physical/chemical stimulus in a specific way, and that can be transformed into a physical quantity or a signal, for measurement and/or monitoring purposes by an instrument (BASSOI et al., 2019).
Digital agriculture, due to the computational support available to users, introduced a new level of technology, which includes robotics, remote sensing, geoprocessing, decision making and statistical processes (BASSOI et al., 2019). Added to this is the emergence of IoT, which allows objects to be controlled remotely through a network, creating a direct integration between the physical world and computer-based systems (LEHMANN et al., 2012). The application of IoT concepts in the agricultural area has great potential for growth, as technologies such as Wireless Sensor Networks (RSSF) and Radio Frequency Identification (RFID) are becoming increasingly accessible and low-cost. Thus, the so-called smart farms (KALOXYLOS et al., 2012) emerge, in which the farmer uses these technologies, associated with mobile location and real-time tracking and monitoring of objects.
Drones
One of the technologies used in precision agriculture is drones, also known as UAVs (Unmanned Aerial Vehicles). (GONÇALVES; CAVICHIOLI, 2021). Initially developed by defense industries, drones have taken on new tasks in other areas, such as agriculture. (MESQUITA, ARIOSTO, 2014).
Drones come in many different models, from the simplest to the most complex, from those that perform multiple functions to those used for leisure. Drones come in three different types, namely: fixed wing drones, multi-rotor drones and single rotor drones. (SENAR, 2018). They are capable of showing us images that we would hardly be able to see because they have high-quality cameras, collecting high-definition images in specific and difficult-to-access places. Drone cameras are divided into two types: RGB and NIR cameras and multispectral cameras. (DRONES AND ENGINEERING, 2020).
With the modernization of the field, drones are increasingly available, whether for small or large producers. According to Bruna Eduarda Meinen Feil (2018), the advantages of using drones in agriculture are: reduced monitoring time of the cultivated area, mapping of areas that are difficult to access, discovery of adversities in the crop (such as pests, diseases and excess or lack of irrigation), measurement of the concentration of water and nutrients in the soil, reduced cost and high-quality images (GONÇALVES; CAVICHIOLI, 2021). Their disadvantages are: restrictions on flight with height limits, operating radius and inappropriate locations such as airports, documentation required to pilot drones and in some multi-rotor drones, the short operating time in the air (MEINEN FEIL, 2018).
In just a few years, drones have been modernized in their entirety and have thus begun to benefit sectors that until then did not have much technology, such as agriculture. They are now more accessible to producers who can purchase or rent equipment, thus increasing crop productivity (GIRALDELI, 2019). According to Bastos (2015), these are the activities that drones perform in the field that deserve to be highlighted: Crop analysis is perhaps the main use of drones in agriculture, detecting pests or diseases, planting failures, excess or lack of irrigation, and analyzing crops through software that captures images.
The drone hovers over the crop, capturing images that are organized chronologically using software, making it possible to check the development of the crop (BASTOS, 2015). Through images captured by drones during their flight and using software, it is possible to take measurements in the field. With the images captured by drones, it is possible to demarcate areas, selecting the best places to plant and even to demarcate water sources and rivers for preservation (figure 2) (GIRALDELI, 2019).
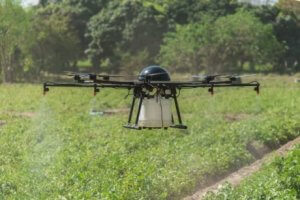
With high technology in drones, their precision has become excellent for spraying in specific places, reducing the cost of chemical products, spraying only the areas in need, reducing the cost of inputs by up to 50% (GIRALDELI, 2019). Learn about the obligations and rules for using drones in agriculture.
According to Ana Lígia Giraldeli (2019), with the use of drones in agriculture, it is also possible to:
- Discover occurrence of fires;
- Deforested areas;
- River sources;
- Recognition of areas with difficult access;
- Estimate productivity;
- Agricultural and water mapping;
- Impose precision agriculture.
Over the years, agriculture has been modernized and gained new tools to assist in production, such as machines, agricultural implements, inputs and drones that were initially used in military activities have entered the agricultural sector and gained new versions, increasingly updated with the capacity for new tasks. New models have been developed, which can be single-rotor, multi-rotor or fixed-wing, and their cameras have become increasingly better, generating images that are difficult to see with the naked eye and even infrared images (GONÇALVES; CAVICHIOLI, 2021).
With all activities carried out with precision, drones have become a fundamental tool in agriculture, with projections of being increasingly present in the field, whether on large or small properties, as it reduces the time and costs for producers, consequently increasing productivity (GONÇALVES; CAVICHIOLI, 2021).
The current trend of rising production costs, reduced labor, increased market demands for safer food, climate change, conservation of natural resources and environmental contamination are factors that have contributed to the intensification of the development of automation and precision agriculture. This scenario has required the implementation of new, increasingly multidisciplinary agricultural practices, in which automation and precision agriculture are key elements. (BASSOI et al., 2019).
References
BASSOI, Luís Henrique et al. Precision agriculture and digital agriculture. TECCOGS: Digital Journal of Cognitive Technologies, n. 20, 2019.
BASTOS, 2015. 15 uses of drones in agriculture and livestock. Available at: https://revistagloborural.globo.com/Noticias/Pesquisa-e-Tecnologia/noticia/2015/05/15-usosde-drones-na-agricultura-e-na-pecuaria.html.
BERRY, JK et al. Precision conservation for environmental sustainability. Journal of Soil and Water Conservation, no. 58, vol. 6, p. 332-339, 2003.
BERRY, JK et al. Applying spatial analysis for precision conservation across the landscape. Journal of Soil and Water Conservation, no. 60, vol. 6, p. 363-370, 2005.
BRETTEL, M. et al. How virtualization, decentralization and network building change the manufacturing landscape: an industry 4.0 perspective. International Journal of Mechanical Engineering and Applications, vol. 8, no. 1, p. 37-44, 2014.
DELGADO, JA, BERRY, JK Advances in precision conservation. Advances in Agronomy, n. 98, p. 1-44, 2008.
DRONES AND ENGINEERING. TYPES OF CAMERAS FOR DRONES AND THE IMAGES GENERATED, 2020. Available at: https://blog.droneng.com.br/tipos-de-cameras-para-drones/.
EZENNE, GI et al. Current and potential capabilities of uas for crop water productivity in precision agriculture. Agricultural Water Management, vol. 218, p. 158-164, 2019.
GIRALDELI, Ana Lígia. Drones in agriculture: how they help you make more profit. Aegro, 2019. Available at: https://blog.aegro.com.br/drones-na-agricultura/.
GONÇALVES, Vinícius Parlangeli; CAVICHIOLI, Fábio Alexandre. Study of the functionalities of drones in agriculture. Technological Interface Magazine, v. 18, no. 1, p. 321-331, 2021.
INAMASU, RY et al. Agricultural, livestock and forestry automation portfolio. São Carlos: Embrapa Instrumentation, 2016. 14 p. (Embrapa Instrumentation. Documents, 60).
INAMASU, RY; BERNARDI, ACC Precision agriculture. In:bernardi, ACC et al. (org.). Precision agriculture: results of a new perspective. Brasília, DF: Embrapa, 2014. p. 21-33.
INAMASU, RY et al. Precision agriculture for the sustainability of Brazilian agribusiness production systems. In: Inamasu, RY et al. (org.). Precision agriculture: a new perspective. São Carlos: Embrapa Instrumentação, 2011. p. 14-26.
KALOXYLOS, A. et al. Farm management systems and the Future Internet era. Computers and Electronics in Agriculture, v. 89, p. 130-144, 2012.
LEHMANN, RJet al. Future internet and the agri-food sector: state-of-the-art in literature and research. Computers and Electronics in Agriculture, vol. 89, p. 158-174, 2012.
LIAO, Y. et al. Past, present and future of industry 4.0 – A systematic literature review and research agenda proposal. International Journal of Production Research, vol. 55, no. 12, p. 3609-3629, 2017.
MEINEN FEIL, Bruna Eduarda. Is it worth investing in the use of drones in agriculture? Mais Soja. Available at: https://maissoja.com.br/vale-a-pena-investir-no-uso-de-drones-na-agricultura/.
MESQUITA, Ariosto. The advancement of drones. Agro DBO, 2014.
PIVOTO, D. et al. Scientific development of smart farming technologies and their application in Brazil. Information Processing in Agriculture, 2017.
Santos, KEL et al. Geostatistics and geoprocessing in decision-making on the use of inputs in a pasture. Brazilian Journal of Biosystems Engineering, v. 11, n. 3, p. 294-307, 2017.
SØRENSEN, CG et al. Conceptual model of a future farm management information system. Computers and Electronics in Agriculture, vol. 72, no. 1, p. 37-47, 2010.
WOLFERT, J.; SØRENSEN, CG; GOENSE, D. A future internet collaboration platform for safe and healthy food from farm to fork. Annual SRII Global Conference, 2014. Proceedings… San Jose, CA, USA: SRII p. 266-273, 2014.
Authors
Agr Eng. Dr. Angélica Schmitz Heinzen
Agricultural Eng. Msc. Carolina Custodio Pinto
Agricultural Eng. Msc. Thiago Stella de Freitas